What matters more ...weighs more.
No uniformly agreed methodology exists to weight individual indicators before aggregating them into a composite indicator. Different weights may be assigned to component series in order to reflect their economic significance (collection costs, coverage, reliability and economic reason), statistical adequacy, cyclical conformity, speed of available data, etc.
Weights usually have an important impact on the composite indicator value and on the resulting ranking, especially whenever higher weight is assigned to indicators on which some countries excel or fail. This is why weighting models need to be made explicit and transparent. Moreover, the reader should bear in mind that, no matter which method is used, weights are essentially value judgments and have the property to make explicit the objectives underlying the construction of a composite.
Commonly used methods for weighting include the following:
Weights based on statistical models:
- Principal components analysis
- Data envelopment analysis
- Regression analysis
- Unobserved components models
Weights based on public/expert opinion:
1. Equal weights
In many composite indicators all variables are given the same weight when there are no statistical or empirical grounds for choosing a different scheme. Equal weighting could imply the recognition of an equal status for all indicators (e.g. when policy assessments are involved). Alternatively, it could be the result of insufficient knowledge of causal relationships, or ignorance about the correct model to apply, or even stem from the lack of consensus on alternative solutions. The impact of equal weighting on the composite indicator also depends on whether equal weights are applied to single indicators or to components (which group different number of indicators).
Weights based on statistical models
In equal weighting it may happen that - by combining indicators that are highly correlated – one may introduce an element of double counting into the index. A solution has often been given by testing indicators for statistical correlation (e.g. Pearson correlation coefficient) - and choosing only indicators having a low degree of correlation or adjusting weights accordingly, e.g. giving less weight to correlated indicators. Furthermore, minimizing the number of indicators in the index may be desirable on other grounds such as transparency and parsimony.
There will almost always be some positive correlation between different measures of the same aggregate. Thus, a rule of thumb should be used, so as to decide on the threshold beyond which correlation entails double counting. If weights should ideally reflect the contribution of each indicator to the index, double counting should not only be determined by statistical analysis but also by the analysis of the indicator itself versus the rest of indicators and the phenomenon they all aim to measure.
2. Principal components analysis/ Factor analysis
Principal component analysis and more specifically factor analysis group together indicators that are collinear to form a composite indicator capable of capturing as much of common information of those indicators as possible. Each factor reveals the set of indicators having the highest association with it. The idea under this approach is to account for the highest possible variation in the indicators set using the smallest possible number of factors. Therefore, the index no longer depends upon the dimensionality of the dataset but it is rather based on the “statistical” dimensions of the data. According to this method, weighting only intervenes to correct for the overlapping information of two or more correlated indicators, and it is not a measure of importance of the associated indicator.
The first step is to check the correlation structure of the data: if the correlation between the indicators is low then it is unlikely that they share common factors.
The second step is the identification of a certain number of latent factors, lower than the number of indicators. Briefly, each factor depends on a set of coefficients (loadings), each coefficient measuring the correlation between the individual indicator and the latent factor. Principal component analysis is usually used to extract factors). For a factor analysis only a subset of principal components are retained (let’s say m), the ones that account for the largest amount of the variance. The standard practice is to choose factors that: (i) have associated eigenvalues larger than one; (ii) individually contribute to the explanation of overall variance by more than 10%; (iii) cumulatively contribute to the explanation of the overall variance by more than 60%.
The third step involves the rotation of factors. The rotation (usually the varimax rotation) is used to minimise the number of indicators that have a high loading on the same factor. The idea in transforming the factorial axes is to obtain a “simpler structure” of the factors (ideally a structure in which each indicator is loaded exclusively on one of the retained factors). Rotation is a standard step in factor analysis, it changes the factor loadings and hence the interpretation of the factors leaving unchanged the analytical solutions obtained ex-ante and ex-post the rotation.
The last step deals with the construction of the weights from the matrix of factor loadings after rotation, given that the square of factor loadings represent the proportion of the total unit variance of the indicator which is explained by the factor. Notice that different methods for the extraction of principal components imply different weights, hence different scores for the composite (and possibly different country ranking).
3. Data envelopment analysis
Data envelopment analysis uses linear programming to retrieve an efficiency frontier and uses this as benchmark to measure the performance of a given set of countries. The set of weights derives from this comparison. Two main issues are involved in this methodology: the construction of a benchmark (the frontier) and the measurement of the distance between countries in a multi-dimensional framework.
The construction of the benchmark is done by assuming:
- positive weights (the higher the indicator value, the better for the corresponding country);
- non discrimination of countries that are best in any single dimension (i.e. indicator) thus ranking them equally; and
- a linear combination of the best performers is feasible (convexity of the frontier).
The distance of each country with respect to the benchmark is determined by the location of the country and its image position in the frontier. Both issues are represented in the following figure for the simple case of 4 countries and only two indicators.
Performance frontier determined with Data Envelopment Analysis
In the figure two indicators are represented in the two axes and four countries (a, b, c, d) are ranked according to the score of the indicators. The line connecting countries a, b and c constitutes the performance frontier and is the benchmark for country d which lies beyond the frontier. The countries on the frontier are classified as the best performing, while country d has lower performance. The degree of performance is defined by the ratio of the distance between the origin and the actual observed point and that of the projected point in the frontier: . The best performing countries will have a performance score of 1, while for the least performing it will be lower than one. The set of weights of each country will therefore depend on its position with respect to the frontier. The benchmark will correspond to the ideal point exhibiting a similar mix of indicators (d in the example).
The benefit-of-the-doubt procedure is a particular case of data envelopment analysis, which allows countries to emphasise and prioritise those aspects for which they perform relatively well (identification of target on the efficiency frontier). The weights, in this approach, are country-dependent and sensitive to the benchmarks. In general, even using the best combination of weights for a given country, other countries may show better performance. The optimisation process could lead to many zero weights if no restrictions on the weights were imposed. In such cases, many countries would be considered as benchmarks. Bounding restrictions on weights are hence necessary for this method to be of practical use.
4. Regression analysis
Linear regression models can tell us something about the 'linkages' between a large number of indicators and a single output measure that represents the objective to be attained. A (usually linear) multiple regression model is then estimated to retrieve the relative weights of the indicators. This approach, although suitable for a large number of indicators of different types, implies the assumption of linear behaviour and requires the independence of explanatory indicators. If these indicators are correlated, in fact, estimators will have high variance meaning that parameters estimates will not be precise and hypothesis testing not powerful. In the extreme case of perfect collinearity among indicators the model will not even be identified. It is further argued that if the concepts to be measured could be represented by a single measure, then there would be no need for developing a composite indicator. Yet, this approach could still be useful to verify and adjust weights, or when interpreting indicators as possible policy actions. The regression model, thereafter, could quantify the relative effect of each policy action on the target, i.e. a suitable output performance indicator identified on a case-by-case basis.
5. Unobserved components models
Weights within the unobserved components models are obtained by estimating with the maximum likelihood method a function of the indicators. The idea is that indicators depend on an unobserved variable plus an error term, e.g. the “percentage of firms using internet in country j” depends upon the (unknown) propensity to adopt new information and communication technologies plus an error term accounting -let’s say- for the error in sampling firms.
Therefore, by estimating the unknown component it will be possible to shed some light on the relationship between the composite and its components. The weight obtained will be set so as to minimize the error in the composite. This method resembles the linear regression approach (although the interpretation is different). The main difference is that the dependent variable is unknown. The weights, in this approach are equal to:
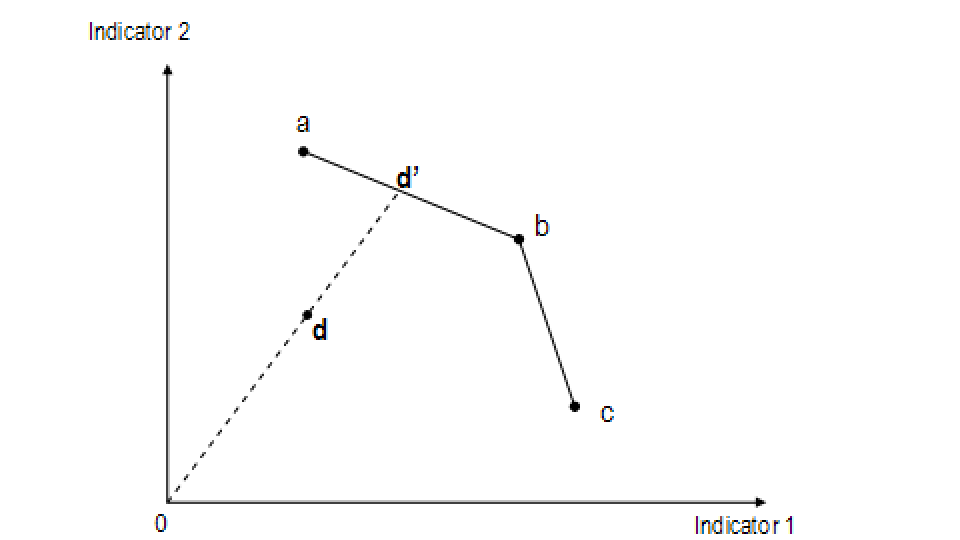
Performance frontier determined with Data Envelopment Analysis
where the weight for country c and indicator q, w (c,q), is a decreasing function of the variance of indicator q (implying that the higher the variance of the indicator, the lower is its precision and the lower the weight assigned to the indicator), and an increasing function of the variance of the other indicators. However, in case of missing data, the sum in the denominator could be made be calculated upon a country-dependent number of elements. This may produce non comparability of country values for the composite indicator. Obviously whenever the number of indicators is equal for all countries then weights will no longer be country specific and comparability will be assured.
Weights based on public/expert opinion
Participatory approaches, which involve public or expert judgement, are often used for the determination of the weights, with a view to express the relative importance of the indicators from the societal viewpoint.
6. Budget allocation
In the budget allocation method experts are given a budget of N points, to be distributed over a number of indicators, paying more for those indicators whose importance they want to stress. The budget allocation method can be divided in four different phases: (a) Selection of experts for the valuation; (b) Allocation of budget to the indicators; (c) Calculation of the weights; (d) Iteration of the budget allocation until convergence is reached (optional). In some policy fields, there is consensus among experts on how to judge at least the relative contribution of physical indicators to the overall problem. There are certain cases, though, where opinions diverge. It is essential to bring together experts that have a wide spectrum of knowledge, experience and concerns, so as to ensure that a proper weighting system is found for a given application. As the allocation of a budget over a too large number of indicators can give serious cognitive stress to the experts, this method is suitable for sets of no more than circa 10 indicators.
7. Public opinion
In public opinion polls, issues are selected which are already on the public agenda, and thus enjoy roughly the same attention in the media. From a methodological point of view, opinion polls focus on the notion of concern, that is people are asked to express “much” or “little concern” about certain problems measured by the indicators. As with expert assessments, the budget allocation method could also be applied in public opinion polls. However, it is more difficult to ask the public to allocate a hundred points to several indicators than to express a degree of concern about the problems that the indicators represent.
8. Analytic Hierarchy Process
The Analytic Hierarchy Process is widely used in multi-attribute decision making. It enables decomposition of a problem into hierarchy and assures that both qualitative and quantitative aspects of a problem are incorporated in the evaluation process, during which opinion is systematically extracted by means of pairwise comparisons, by firstly posing the question “which of the two indicators is more important?” and secondly “by how much?”. The strength of preference per pairs of indicators is expressed on a semantic scale of 1 (equality) to 9 (i.e. an indicator can be voted to be 9 times more important than the one to which it is being compared). The relative weights of the indicators are then calculated using an eigenvector technique, which allows to resolve inconsistencies, e.g A better than B better than C better than A loops.
9. Conjoint analysis
Merely asking respondents how much importance they attach to an indicator is unlikely to yield effective “willingness to pay” valuations. Those can be inferred by using conjoint analysis from respondents’ ranking of alternative scenarios.
The conjoint analysis is a decompositional multivariate data analysis technique frequently used in marketing and consumer research. This method asks for an evaluation (a preference) over a set of alternative scenarios (a scenario can be thought as a given set of values for the indicators). Then this preference is decomposed by relating the single components (the known values of indicators of that scenario) to the evaluation.
Although this methodology uses statistical analysis to treat data, it operates with people (experts, politicians, citizens) who are asked to choose which set of indicators they prefer. The absolute value (or level) of indicators would be varied both within the choice sets presented to the same individual and across individuals. A preference function would be estimated using the information coming from the different scenarios.
Therefore a probability of the preference could be estimated as a function of the levels of the indicators defining the alternative scenarios. After estimating this probability (often using discrete choice models), the derivatives with respect to the indicators of the preference function can be used as weights to aggregate the sub-indicators in a composite index.
Next
Originally Published | Last Updated | 21 Apr 2018 | 01 Dec 2020 |
Knowledge service | Metadata | Composite Indicators |
Share this page